Artificial Intelligence (AI) plays a key role in the modern automotive industry, transforming both manufacturing processes and the vehicles themselves. Not only does it enable the optimisation of component manufacturing by reducing time required and waste generated, it also improves quality and production management.
The impact of AI on production processes in the automotive industry
Artificial Intelligence brings innovative solutions to the automotive industry and significantly improves the efficiency of manufacturing companies. One of its manifestations is the increasing automation and thorough quality control of components. Intelligent robots carry out their assigned, often repetitive, tasks with a high degree of precision. This can include parts assembly or welding and painting. Machine learning in manufacturing also supports companies in forecasting their raw material consumption, leading to a reduction in waste and associated costs. Artificial intelligence analyses historical data on material consumption and, based on this, predicts future demand. Intelligent algorithms are also able to identify areas of material loss and introduce production optimisation.
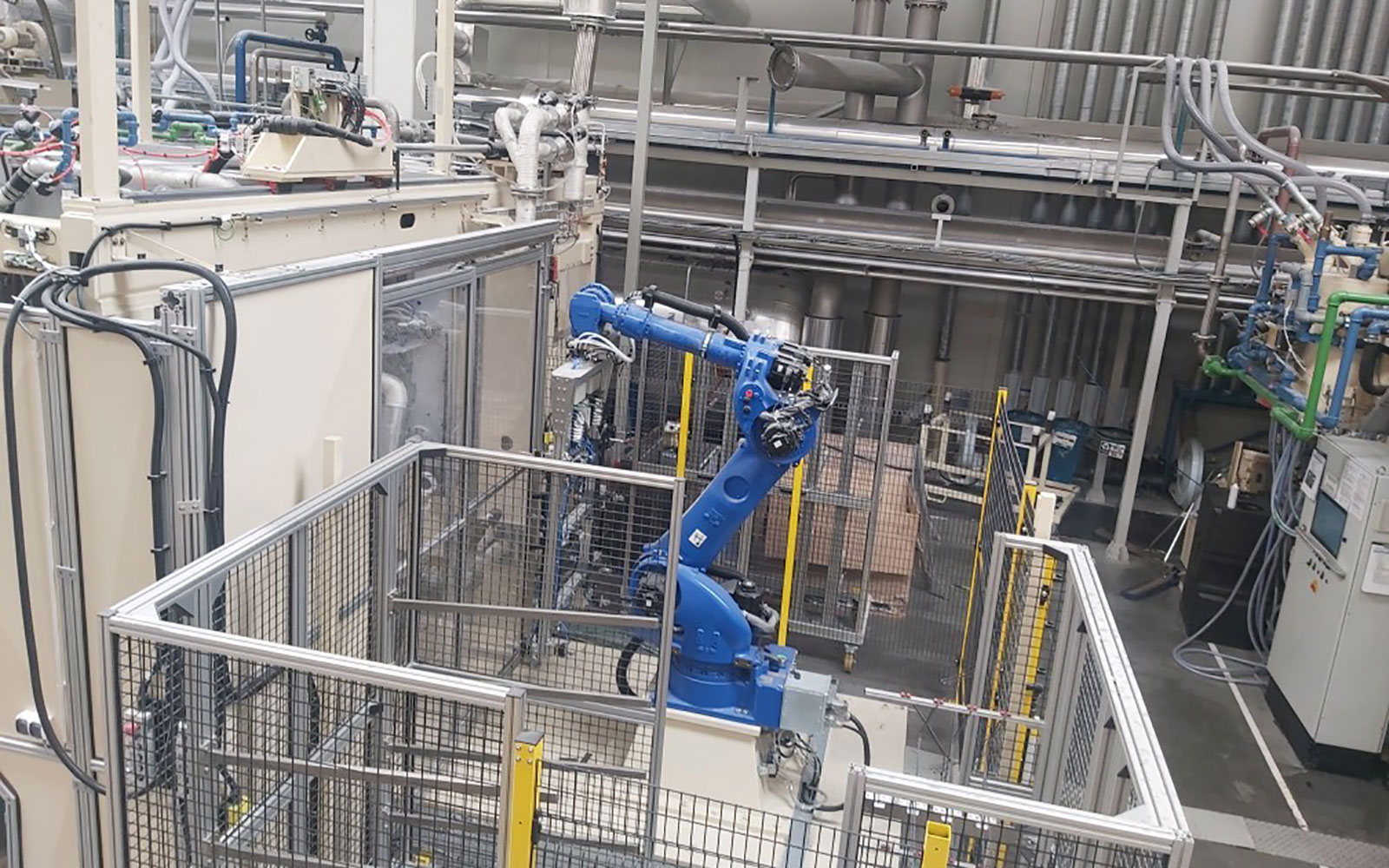
AI is also often responsible for maintaining production continuity these days. It monitors the performance of production machines in real time by analysing data received from sensors, such as vibration, operating temperature or load. On this basis, the system detects potential problems before failure occurs. With predictive analytics, artificial intelligence plans maintenance work in such a way as to minimise downtime and the associated costs. AI also plays a key role in the area of quality control, performing visual inspections, analysing camera images in real time and identifying even microscopic defects. This significantly increases the reliability and durability of the components and minimises any potential complaints.
Key areas for optimisation in automotive component production
The optimisation of production processes is key to increasing manufacturing efficiency, reducing costs and improving quality, thereby increasing the competitiveness of automotive companies. As we already know, there are many areas where artificial intelligence and the latest advances in Industry 5.0 can support modern automotive companies. Among the most important aspects from the point of view of production efficiency are planning, quality control, optimisation of material consumption and predictive maintenance.
Production planning
AI enables production process mapping and optimising schedules in a very efficient manner. This eliminates downtime, increases resource efficiency and adapts production to changing demand. Algorithms monitor production processes in real time, identifying any bottlenecks and predicting potential delays. With machine learning, systems can automatically direct resources elsewhere or reorganise tasks to make the best use of production potential. AI analyses the availability of raw materials, machinery and workers, allocating them to production areas based on current needs. Algorithms can also predict when specific machines will be needed, maximising energy efficiency.
A very useful function of AI in manufacturing is the intelligent adaptation of manufacturing to current demand. To do this, the algorithms use historical, seasonal and market data, allowing them to anticipate changing customer needs. Based on forecasts of increased or decreased demand, AI automatically changes production schedules. For example, if a component manufacturer suddenly receives a large order, AI can analyse the availability of raw materials and machinery, generate a new schedule and allocate resources differently or increase the staffing of relevant production positions.
Quality control and defect reduction
In the age of automated production processes, visual inspection of automotive components is an essential part of product quality control, characterised by high precision, speed and being completely maintenance-free. Computer vision systems analyse images of component surfaces with a high degree of accuracy, identifying any micro-cracks, scratches or other defects that may be invisible to the human eye and, in the long term, become the cause of potential failure.
Thanks to special cameras and sensors, quality control takes place in real time, which optimises production processes. These systems use deep learning models (e.g. neural networks) and automatically classify parts as defective or compliant. Based on large data sets, the algorithms are able to recognise different types of defects. Furthermore, the algorithms responsible for quality control can be integrated with industrial robots that automatically remove defective parts from the production line. AI not only detects and rejects defective parts, but can also prevent defects from occurring in the future. It collects and analyses data from various stages of production to identify the source of the problem (e.g. a faulty material or an error in the production process) and then recommends preventive measures. The system also generates reports to help engineers implement any measures to optimise production processes.
Optimisation of material consumption
Artificial Intelligence (AI) algorithms contribute significantly to the more efficient use of raw materials in production processes, leading to, among other things, a reduction in waste. AI uses advanced algorithms that indicate how to optimally cut materials so that losses are minimised. The systems also monitor production processes in real time, identifying where waste is occurring and immediately suggesting corrective action.
By using AI in production, potential opportunities for recycling waste can also be analysed, further reducing the amount of unused raw materials in the process. With machine learning, the algorithms can also check historical data related to processing parameters. On this basis, they adjust the operation of the machines to maximise productivity and production quality. Intelligent systems can dynamically adjust machine settings in response to changing conditions, such as differences in raw material parameters. AI also predicts the efficiency and performance of the process and anticipates potential problems before they arise.
Predictive maintenance
AI is changing the way sensor data from production machines is analysed today, helping to improve reliability and optimise production processes. The IoT (Internet of Things) sensors installed in the machines monitor various variables such as temperature, pressure, vibration or energy consumption. AI analyses this data in real time and identifies deviations from patterns, which can in turn indicate potential failures or anomalies. In some cases, AI can also directly modify the machine's settings, such as reducing the load to avoid overloading.
Conversely, when AI detects a failure, it generates alerts, allowing corrective action to be taken quickly, before the problem affects the entire production process. These systems are often integrated with Management Execution Software (MES), which enables an immediate response when problems are detected. Some advanced AI systems can also simulate failure scenarios, allowing preparation for real-life situations. The most important task of the algorithms, however, is first and foremost to prevent unplanned downtime, including through machine maintenance carried out when needed and efficient planning of the resources needed for maintenance. All of this maximises the impact of maintenance on production.
Examples of AI implementations in automotive component manufacturing
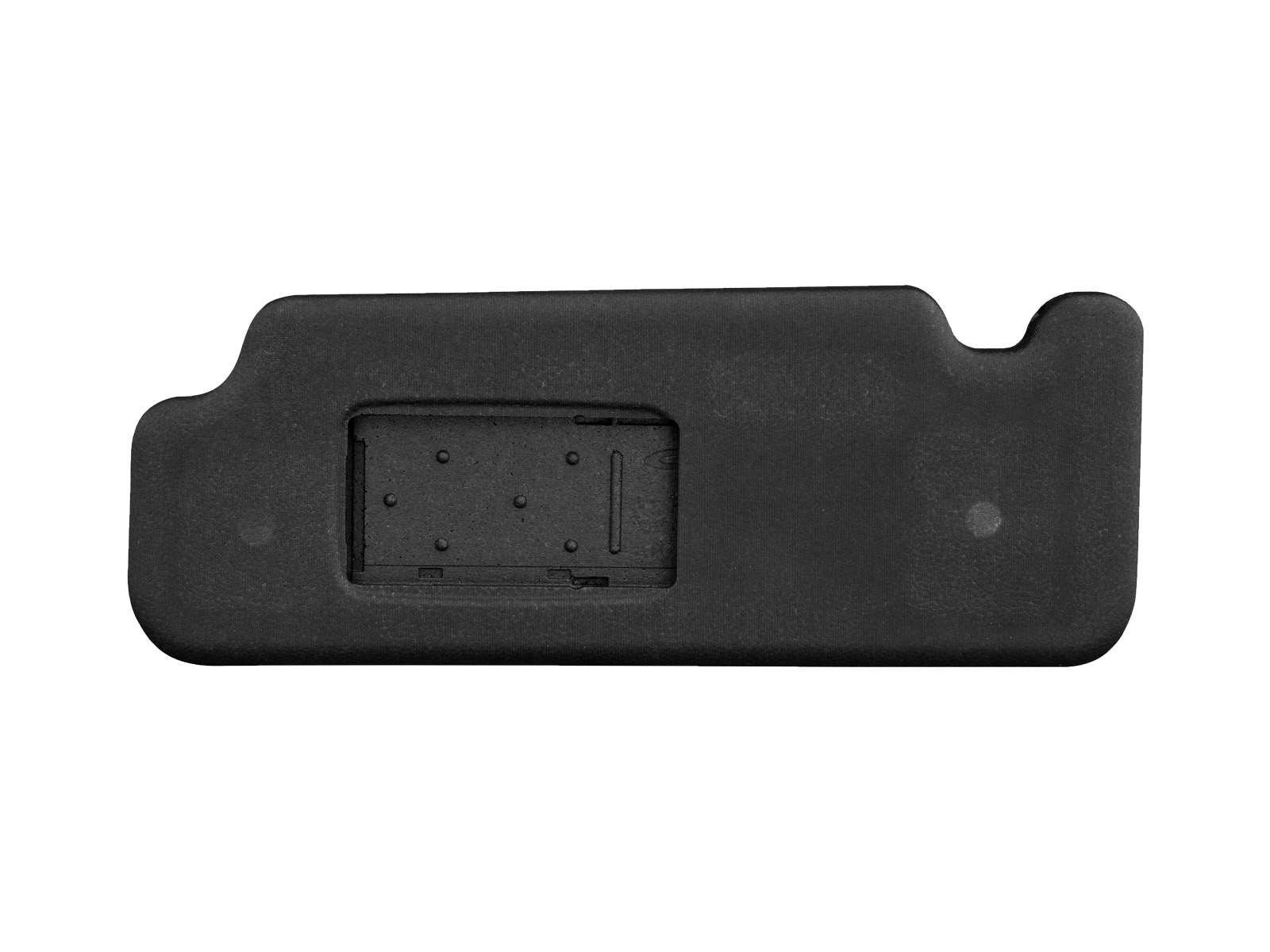
Although the use of AI in manufacturing processes is still new and developing rapidly, many companies are already reaping tangible benefits from integrating intelligent algorithms into their processes. At Knauf Industries Automotive, for example, we have been able to achieve high-precision manufacturing and minimised tolerance deviations for EPP and EPS automotive components, such as expanded polypropylene (EPP) sun guards and transport packaging, among others. Also Plastic Omnium Auto Exteriors, one of the bumper manufacturers for European OEMs such as Opel and Skoda, uses AI to optimise its production processes, reducing waste and improving efficiency. Volkswagen uses AI in its factories to analyse data from sensors in the machines, enabling predictive maintenance and minimising downtime. Ford, on the other hand, is using AI to automate manufacturing processes such as welding and assembly and to accurately analyse production data to detect problems faster and improve product quality.
While the specific benefits of implementing AI may vary from company to company and area to area, data collected from leading manufacturing facilities using the technology shows that by using AI to optimise material cutting and forming processes, waste can be reduced by up to 20-30%. Meanwhile, through automation and better resource management, production efficiency can increase by 15-25%, and AI-assisted visual inspection reduces defects by 10-20%.
Implementing AI in manufacturing – where to start?
Implementing AI to optimise production processes is a complex but extremely fascinating process. The basis for this is first and foremost a thorough analysis of current processes. It is important to check which ones are time-consuming, costly, error-prone or worth improving. In this context, it is worth identifying areas where efficiency or quality can be improved precisely with the support of AI. It is useful to involve both operational and technical teams in the process, so that needs can be viewed from different perspectives. The next stage is the selection of appropriate AI technologies and tools. It should be based on clearly defined business objectives, such as improving productivity, reducing costs or increasing product quality, for example. This should be followed by an assessment of the available AI tools and platforms in terms of their functionality, ease of integration with existing systems and resource requirements. In order to test them effectively, it is necessary to collect historical and current data from which AI will generate the necessary responses. The testing phase is essential, so it is worth starting with small pilot projects in selected areas to minimise risks and assess the real benefits of using AI. In doing so, the algorithms should be tested on real data and monitored in real time.
Individual solutions are best implemented in stages, starting with those processes that have the greatest impact on overall production efficiency. Finally, it is worth remembering that the implementation of AI solutions is only the beginning. These processes should be monitored regularly and modifications implemented on an ongoing basis to improve the models. To this end, the opinions and insights of employees who work with AI technologies on a daily basis will be helpful.