Machine Learning technology is mainly talked about in the context of self-driving cars. However, the technology is also finding increasing use in automotive manufacturing, not only improving product quality, but even helping to streamline entire production lines.
Machine Learning – what is it?
Machine Learning (ML for short) is a branch of Artificial Intelligence based on the idea that systems can learn from data, identify patterns and make their own decisions on that basis. Machine Learning algorithms construct a mathematical model based on sample data (the so-called training set) for prediction or classification. In practice, this means that machines 'learn' and can make decisions on their own without human intervention.
This mechanism is divided into three main stages, which are the decision-making process, the loss function and model optimisation. In the first phase, ML algorithms classify data or predict performance outcomes based on input data. The result is an estimate of the pattern contained in the data. At the loss function stage, the quality of the model is assessed. If sensor or camera readings are available, the model results are compared with the actual data to assess its accuracy. The final step is model optimisation. If the model can better fit the actual data, an adjustment is made to minimise the differences between the model estimate and the actual data. The algorithm repeats this iterative 'evaluation and optimisation' process until the required level of precision is achieved.
Machine Learning in automotive quality control
The streamlining of production processes is key to ensuring high product quality and manufacturing efficiency, particularly in microfactories the automotive industry. Machine Learning plays a key role in the area of quality control in the processes of image analysis, failure prediction and even complex production line streamlining. Vision systems analyse images of components detecting defects such as cracks, chips or uneven spots. This allows defective products to be automatically rejected. Specialised cameras and software are used to assess the quality of the components with a high degree of accuracy. Machine vision analyses the parameters of automotive parts such as dimensions, shape or finish. Vision systems can also read barcodes or QR codes, verifying their correctness. This allows products to be identified and their history to be tracked. Code reading is a key element in the area of robot control in smart factories, which can thus carry out, for instance, the assembly of components or the transport of materials from the warehouse to the production line. This way, they can work effectively with humans – in line with the Industry 5.0 concept. Machine Learning algorithms further analyse sensor data to predict potential machine failures. This enables planning maintenance activities to minimise unplanned downtime and maintain production continuity. Furthermore, Machine Learning analyses data from past failures, identifying patterns and risk factors for the future. ML enables not only the avoidance of problems, but also the streamlining of car design and production processes, e.g. through the reorganisation of production lines, the implementation of modern technologies or performance analysis. As a result, Machine Learning contributes comprehensively to increasing quality, efficiency and safety in the area of automotive parts production.
Application of Machine Learning in automotive parts manufacturing
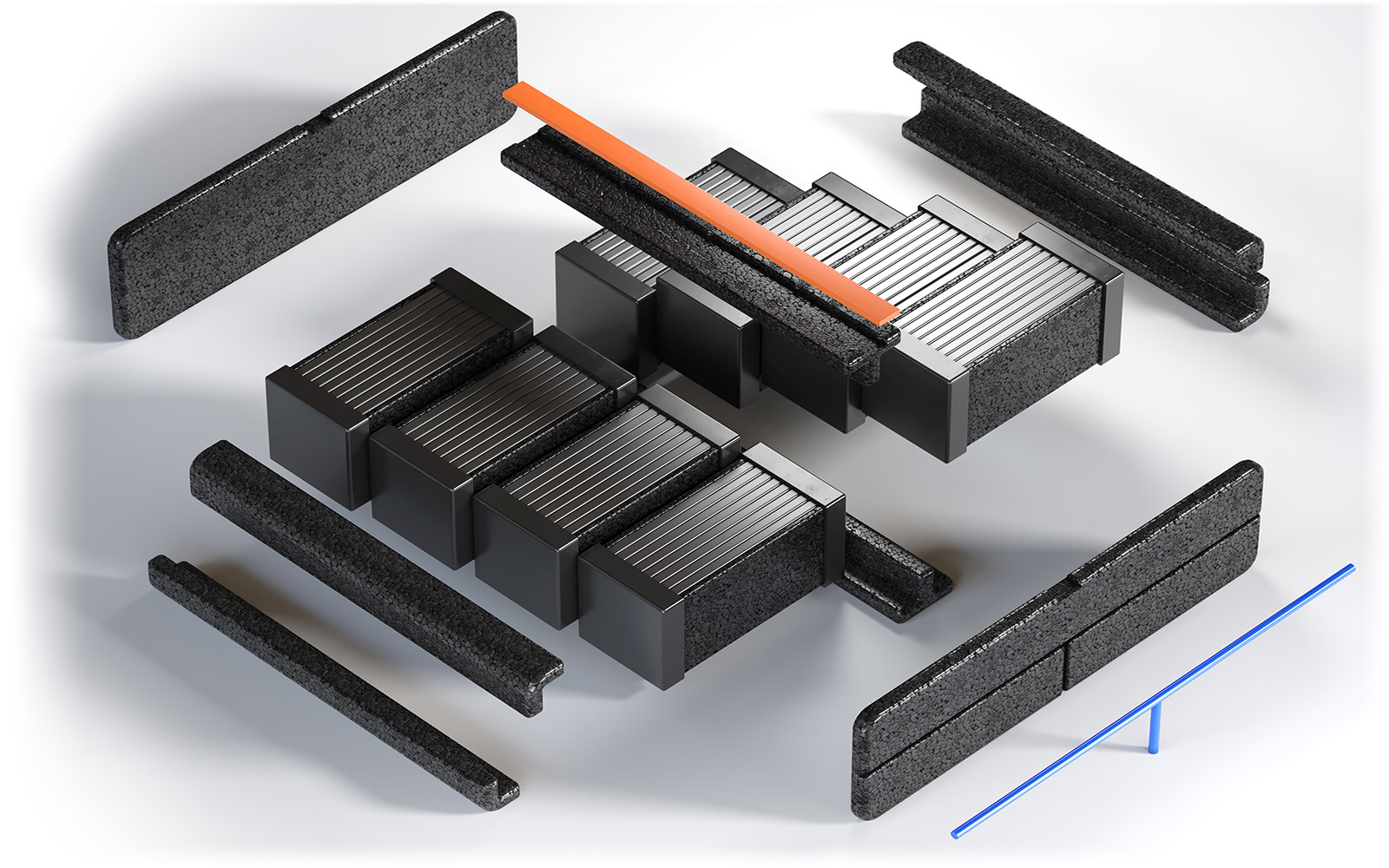
ML algorithms are not only used to analyse data from machines and production processes, thus minimising downtime and reducing repair costs. They allow more data to be analysed and patterns to be recognised, leading to cost and efficiency optimisation at various stages of vehicle production, from planning activities to logistics processes. Artificial Intelligence can help, for example, to better align production with demand, eliminate waste and increase manufacturing flexibility in line with the concept of lean manufacturing. It can, for example, adapt the options, colour or layout of the interior to customers' preferences. ML is an integral tool for improvement, resulting in greater competitiveness and efficiency for companies in the automotive industry. Thanks to the automation of production processes, CNC (computer numerically controlled) machines make it possible to manufacture even the most complex automotive parts, such as seat components, battery housings or dashboards. Thanks to special software, they ensure precision, accuracy and reproducible quality during mass production and, above all, compliance with technical specifications. In summary, Machine Learning contributes to optimizing car manufacturing to the highest standards.
Benefits of Machine Learning in the automotive industry
The application of Machine Learning technology in the automotive industry has multiple advantages. Machine Learning technology enables efficient processing of large amounts of data, leading to faster and better decision-making. Furthermore, ML algorithms identify patterns and make predictions based on historical data, making accurate decisions on their own, reducing the need for human supervision. They are able to analyse vast amounts of information that a human could not possibly grasp with their mind. This is particularly useful for organisations processing large data sets. Above all, however, ML carries the potential for cost savings. Automating processes leads to lower costs, relieves the burden on employees and increases their safety. In this way, Machine Learning technology contributes to competitiveness, efficiency and innovation in the automotive industry. The application of Machine Learning in the quickly developing area of self-driving vehicles (so-called AutoAI or AutoML) should also not be forgotten. AutoML algorithms learn to respond to changing traffic conditions, improving the safety of road users. In the future, they may even lead to a change in the way we travel.